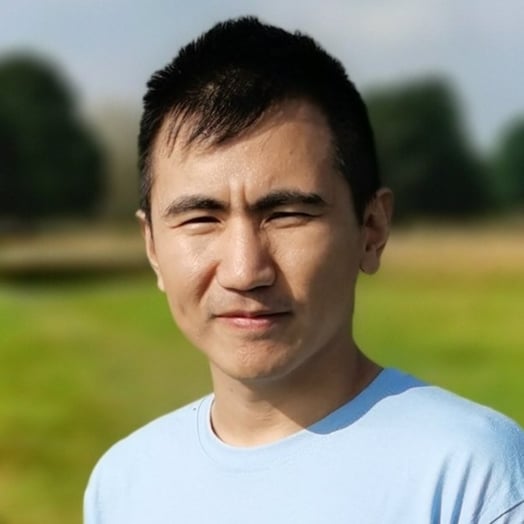
Chen Jin
Verified Expert in Engineering
Computer Vision Developer
Jin has multidisciplinary experience developing deep learning and computer vision methods for medical, auto-piloting, and geospatial image sectors, with works published at ICLR, NeurIPS, and MICCAI. He developed data-and-computation-efficient machine learning methods for budgeted computer vision tasks such as segmentation, detection, classification, super-resolution, GAN, and 3D reconstruction. Jin also excels at multimodal self-supervised representation learning, image generation, and mapping.
Portfolio
Experience
Availability
Preferred Environment
Python, PyTorch
The most amazing...
...我开发的方法是用于分割的自适应降采样, 它提高了10%的精度,同时比SoTA节省了90%的计算.
Work Experience
多模态学习与图像生成研究员
University College London
- Developed a multimodal-multiscale MRI-histology-text self-supervised representation learning method and bypassed the need to use expensive and destructive histology images for the first time.
- 创建了一个跨模态尺度的mri -组织学-文本图像生成和映射(高档1),000 times) method for explainable AI.
- Developed an interactive web UI based on a Gradio API to showcase registered cross-modality image patches.
- Co-developed a disentangling inter-reader variability in segmentation method and published it at MICCAI/NeurIPS 2020.
Researcher
University College London
- Developed an attention-based deformable downsampling method for an end-to-end optimization of low-cost segmentation, 提高了10%的精度,同时比SoTA节省了90%的计算, 并在ICLR 2022上发表了一篇论文.
- Created an attention-based learnable "patch loader" to sample the best size-resolution trade-off configuration at each location for optimal segmentation accuracy, achieved SoTA, 并在MICCAI 2020上发表了一篇论文.
- 参与了三次超高分辨率图像分割挑战, 开发和部署各种SoTA方法, 进入了所有挑战的前10%.
- 对高分辨率十亿像素图像进行高效分割.
Researcher
University College London
- 共同开发了在MIDL 2022和MICCAI 2022上发表的半监督伪标记方法.
- 利用深度学习为癌症成像做出了贡献. 开发了一种用于廉价MRI单模态推理的自监督表示学习方法.
- Hosted the MedICSS 2021 Summer School project and taught a one-week deep learning course for segmentation, including theory and coding, led a team of seven researchers, 在14个团队中获得项目前三名.
Developer and Supervisor
University College London
- Supervised one PhD student working on deep multiple instance learning (MIL) for histological image classification, 谁的最新作品提交给了期刊.
- Supervised and supported two MSc students performing CNN-based segmentation and detection on histology images. One obtained distinctions.
- 开发了一种多尺度循环超分辨方法. Supervised and provided technical support to two MSc students working on super-resolution with CNN and GAN-based methods. Both obtained distinctions.
Research Associate
Heriot-Watt University
- Acted as the research associate working on computational geoscience and 3D modeling of porous media.
- 开发了一种基于滤波器组的弱监督迭代卷积网络, AdaBoost, and auto-context, 提高细线状裂缝的分段连通性. 它在2016年和2018年的会议上提出.
- Contributed to the CT and SEM images registration and multiscale data fusion 并利用多点统计方法指导三维孔隙结构重建 conditional to neighboring "patch."
- 开发了一种基于随机森林的基于斑块的岩石模式自动分类程序.
- Completed the modeling and numerical simulation of macroscale reservoir multiphase flow and presented it to the industry.
Engineering Intern
Canadian Natural Resources Limited (CNRL)
- 基于Eclipse建立油藏流体流动模拟模型.
- 通过模拟研究了各种地质层状构造的影响.
- Performed an analysis of a zonal allocation and potential reservoir in the south Ninian area and presented it to a development team that helped with drilling decisions worth millions.
Experience
超高分辨率图像的下采样分割
http://lxasqjc.github.io/learn-downsample.github.io/作为主要作者,我全权负责开发工作. This work was published at ICLR 2022.
百万像素组织学图像的注视点分割
http://chenjin.netlify.app/publication/foveation/这项工作发表在MICCAI 2020上,我是监督开发的主要作者.
超高分辨率图像的注视点分割
http://chenjin.netlify.app/publication/foveation_ultrahigh/作为监督整个开发过程的主要作者,我在Arxiv上发表了这项工作.
多模态,多尺度,多任务表示学习,图像生成和映射
•开发了一种自我监督的MR组织学文本表示学习方法, 目标是廉价的MRI单模态推断.
•创建了跨模态尺度的MRI组织学图像生成和映射(升级1),000 times) for explainable AI.
• Developed an interactive web UI based on a Gradio API to showcase registered cross-modality image patches.
• Disentangled inter-reader variability in segmentation and co-authored the MICCAI/NeurIPS 2020 publication.
癌症成像分析的分割、分类、检测、超分辨率和GAN
• Supervised a PhD student on deep multiple instance learning (MIL) for large image classification and submission to journals.
• Supervised two MSc projects performing CNN-based segmentation and detection of histology images.
•开发和监督两个基于CNN和gan方法的超分辨率硕士项目.
多尺度模态图像融合、重建和流体模拟
•开发了基于滤波器组的弱监督迭代卷积网络, AdaBoost, 自动上下文,提高细线状裂缝的分段连通性. 它在2016年的会议上展出,并在2018年以海报的形式展示.
• Conducted CT and SEM image registration, multiscale data fusion, 并利用多点统计方法指导三维孔隙结构重建, conditional to neighboring "patch."
• 开发了一种基于随机森林的基于斑块的岩石模式自动分类程序.
• Conducted modeling and numerical simulation of a macroscale reservoir multiphase flow presented to the industry.
医学图像分析挑战- Gleason19
http://gleason2019.grand-challenge.org/•编写R脚本,将六个专家的像素注释合并为一个.
• Developed and trained deep learning models for the segmentation and classification of prostate cancer Gleason grading on ultra-high-resolution histopathological tissue microarray (TMA) images.
• Developed evaluation codes to run on the test dataset as per the task request and submitted them to the competition. Entered the top ten on the leaderboard.
医学图像分析挑战赛- DigestPath2019
http://digestpath2019.grand-challenge.org/• Developed and trained a segmentation model for ultra-high-resolution histology image segmentation and classification.
• Handled the preprocessing of ultra-high-resolution histology images into paired patches suitable for a deep learning model.
•开发评估代码,并将其打包在Docker容器中提交.
地理空间图像分析挑战-远程人工智能
•包括遥感图像数据清洗和预处理.
•部署各种细分模型,以平衡性能效率, such as U-Net, MobileNet, and HR-Net.
•实现各种优化技巧,以提高性能, such as augmentation and regularization.
•根据组织者的测试要求开发测试代码和测试包.
•进入决赛(前10%).
Reservoir Simulation Based on Eclipse
Skills
Languages
Python, Batch, C, R, C++, JavaScript
Libraries/APIs
PyTorch, TensorFlow, Keras
Tools
木星,集群,ImageJ, TensorBoard, MATLAB, ParaView, Cinema 4D
Platforms
Linux, Docker, Eclipse
Other
Computer Vision, Machine Learning, Artificial Intelligence (AI), Image Processing, Deep Learning, Algorithms, Computer Vision Algorithms, Convolutional Neural Networks (CNN), Health, Signal Processing, Models, Cloud, 3D Image Processing, Artificial General Intelligence (AGI), Team Leadership, Object Detection, Object Tracking, Geology, Simulations, Medical Imaging, Satellite Images, Data Representation, Natural Language Processing (NLP), User Interface (UI), Web UI, Gradio, Engineering, Formation Evaluation, Economics, Test Analysis, Calculus, Linear Algebra, Chemical Engineering, Hydrology, Fluid Dynamics, Civil Engineering, Oil & 采购产品天然气,管道,存储,GPT,生成预训练变压器(GPT),提示工程
Paradigms
Data Science
Industry Expertise
Petroleum Engineering
Education
石油工程-计算地球科学博士
赫瑞瓦特大学-英国苏格兰爱丁堡
Master's Degree in Petroleum Engineering
赫瑞瓦特大学-英国苏格兰爱丁堡
石油工程学士学位
中国石油大学(青岛
Certifications
CS231n: Deep Learning for Computer Vision
Stanford University
CS221人工智能:原理与技术
Stanford University
How to Work with Toptal
Toptal matches you directly with global industry experts from our network in hours—not weeks or months.
Share your needs
Choose your talent
Start your risk-free talent trial
Top talent is in high demand.
Start hiring